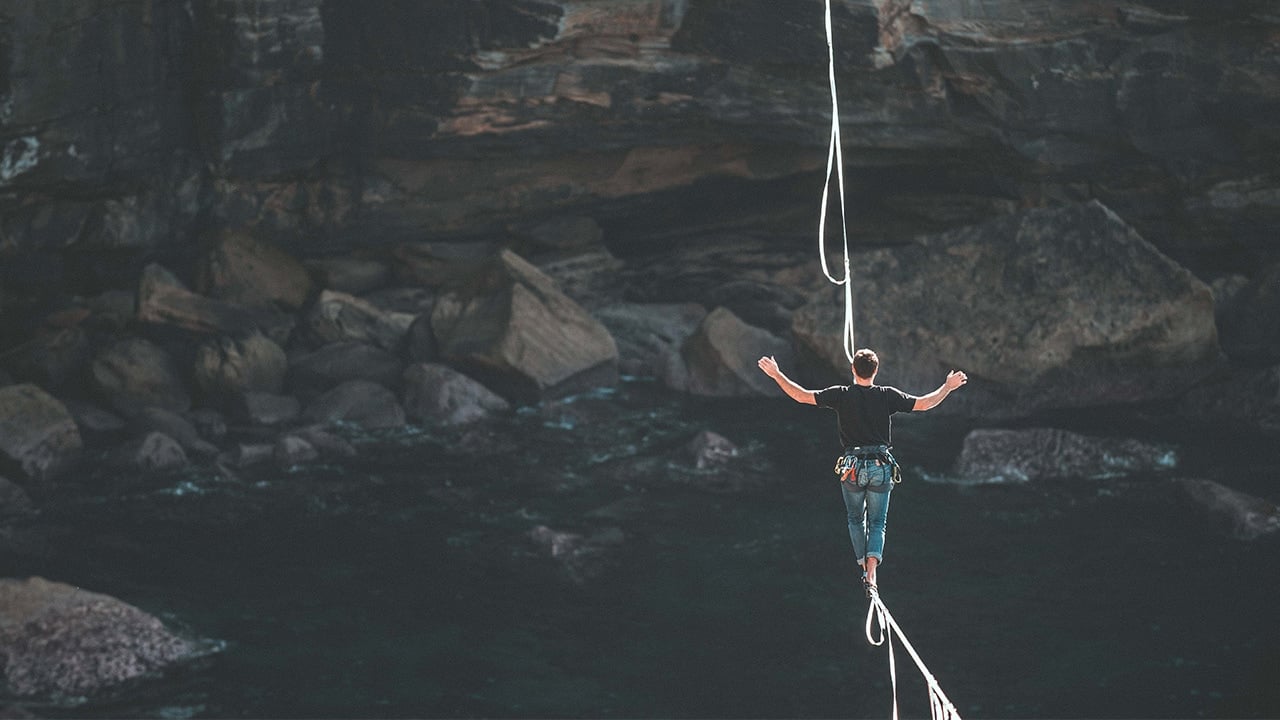
By Shaun Hodgkiss
During a Forbes CEO conference in October 2019, Alibaba co-founder Jack Ma highlighted the importance of “being human” in an age of AI and stressed the importance of teaching skills like painting, sports, dancing, and music to children.
Three years later and you couldn’t swing a bag of cats without hitting a discussion about the controversies behind tools like Stable Diffusion winning painting competitions, ChatGPT writing passable postgraduate theses, and BanterAI impersonating celebrity voices.
However, today’s article is neither a digital witch hunt on innocent software algorithms nor about providing you with insight to the startling improvement in your child’s creative writing grades. Instead, I wanted to pause for a moment to consider the current applications of AI and the potential future of AI in banking and finance.
Chatbots and virtual assistants have been around for a while now, often sitting on the bottom right corner of a website, asking whether they might be able to help. There were many different implementations with varying degrees of complexity, but for the most part are just multiple-choice queries with linear decision paths.
Those tools answer common questions quickly without the need for a human to get involved. The underlying problem with these solutions was that the chatbot would struggle with questions outside its current preset repertoire. There’s very seldom any Artificial Intelligence at play.
Modern AI and machine learning have introduced previously foreign capabilities: context-sensitivity. Chatbots, virtual assistants, and robo-advisors can interact with customers, answer financial queries on personal balances or loans, and provide personalised recommendations. Natural Language Processing (NLP) allows these systems to interpret and respond to customer inquiries.
AI can even supply investment advice, create personalised portfolios, and rebalance investments based on individual financial goals, risk tolerance, and real-time market conditions and trends. Of course, the success of that advice has yet to be tested over the long term, and while we know that even for humans this is often more art than science, we also know how successfully AI can impersonate art.
AI does what AI does best in a similar way for banks. They can use Artificial Intelligence to predict, assess, and manage risks of investments and loans by analysing vast amounts of data. To create a credit score for a customer, AI models can process all kinds of information, from the obvious ones, like transactions and credit history, to more unexpected sources such as social media profiles and online behaviour.
Critics would note that the more intrusive the analysis, the more it raises ethical and privacy concerns – a topic worth its own article. Financial institutions must ensure transparency, fairness, and data protection. The problem here is not the use of AI, but the nature of the collected data. To be fair to AI, human beings might be less efficient at intrusions into our private world, but that doesn’t mean they’re not also effective. And to some degree I would feel less judged by AI browsing through my timeline than another human.
With access to transaction data, AI can be trained to detect patterns indicative of fraudulent activities and help prevent unauthorised transactions, identity theft, terrorist financing, and money laundering.
Using AI to protect your institution and customers has added benefits like enhanced regulatory compliance and fewer false positives.
AI can be used to summarise financial data for reports and to aid financial institutions in automating compliance processes, ensuring adherence to regulations, and generating accurate reports more efficiently and less prone to human error.
You probably noted a couple of common threads in the activities described above. Correctly modelled algorithms can analyse and process data and return results at a speed our human brain can only dream of – nothing surprising there. Financial forecasts based on historical data and linear regression have been zipping through Excel spreadsheets for decades.
The novel part is where NLPs like ChatGPT are taking over a task previously exclusive to humans: Communicating the interpretation of those results in easily understandable language.
Do we have to ask ourselves the click-baity question: “Will AI replace analysts and investment consultants?” Here’s a better idea: Let’s ask ChatGPT, instead!
Compared to AI, the reassurance of talking to another human being who understands the anxiety of safeguarding one’s livelihood is compelling. An investment banker with their own retirement savings in their own fund is a well-practiced model because they have “skin in the game”. What does a computer have to lose if they’re wrong?
On the other hand, who says that empathy could not be modelled into an algorithm? After all, empathy is essentially our interpretation of someone’s emotional state based on a limited dataset consisting of our life experience and what we know about the person next to us. Can we tell the difference between real and feigned empathy? Think back on all the conversations you’ve had with a good friend, and you’ll agree that often we can’t, even when we know the person intimately!
Will the human element remain a vital part of our industry, or is ChatGPT trying to lull us into a false sense of security? Only time (or possibly Jack Ma) will tell.
By Shaun Hodgkiss